Hello,
The concept of a matching engine fascinates me. It is the part of an exchange that determines how orders are matched when a user places an order. The speed and quality of a matching engine determine how often people trade on the exchange it powers. If an engine lags, inefficiencies will creep into the market. For instance, traders running bots may be unable to place orders fast enough, or there may not be enough liquidity for large orders.
I like the concept because matching engines process data from the buy and sell side millions of times daily. And in doing so, they generate a portion of the trade volume in revenue for the exchange. Scaling matching engines enables orders from all kinds of traders to come through and settle without hiccups. Think of them as traffic lights for modern-day financial transactions. Platforms on the web can be imagined as matching engines.
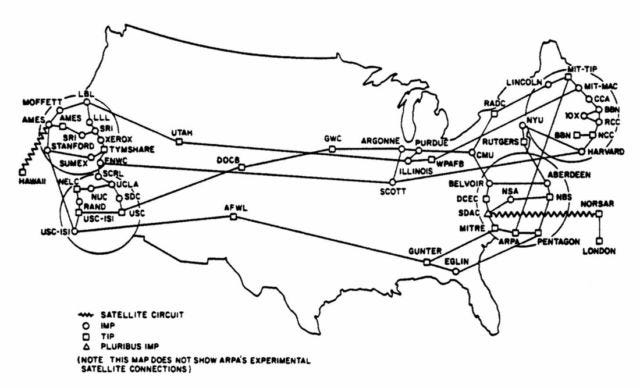
The internet is a graph that maps out content and services. In the 1980s, this graph consisted of a handful of universities and defence research personnel, as shown in the visual above from Arpanet. As the number of participants on this graph scaled, we needed mechanisms to recommend and match queries with the right participant.
Search engines like Google are ‘matching engines’ that match a user’s intent with relevant information on this graph. There is tremendous value in becoming a matching engine that recommends the right content, and that value is reflected in Alphabet’s market capitalisation.
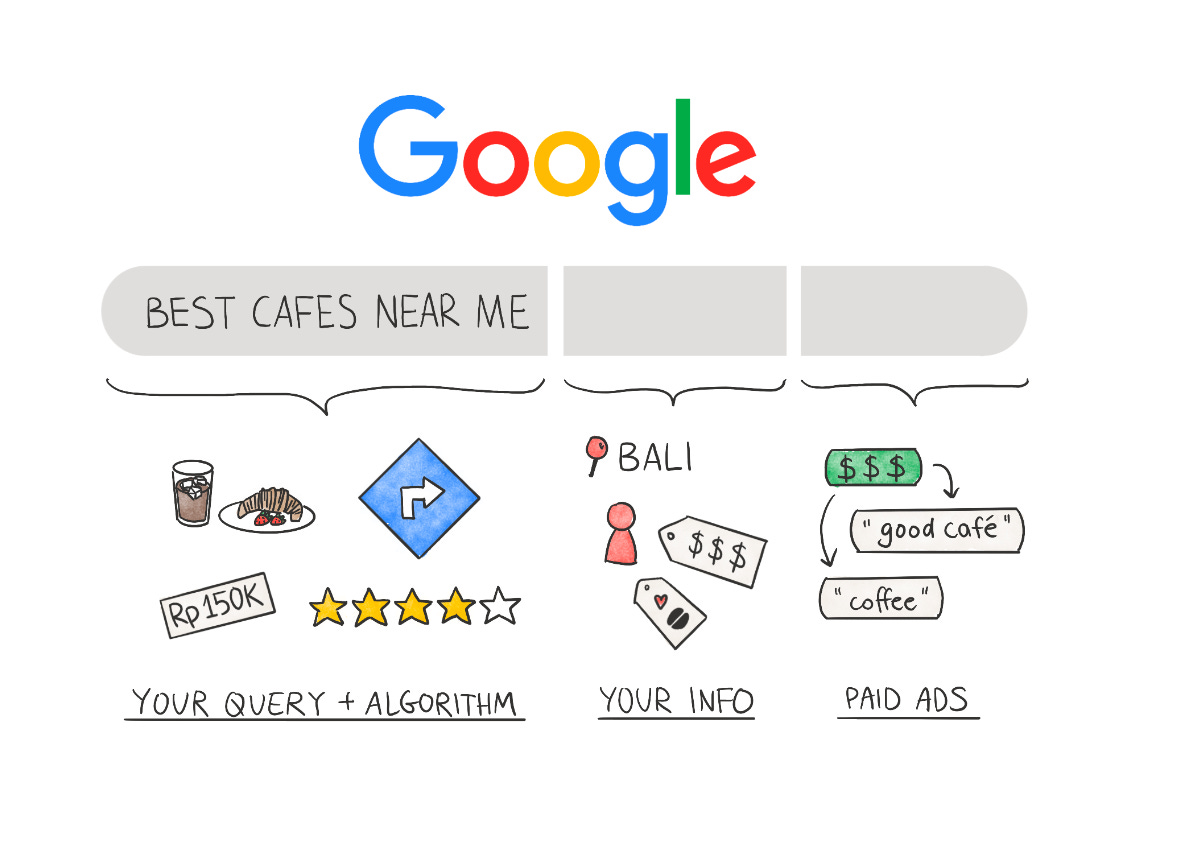
You search Google for ‘best cafes near me’, which becomes the equivalent of a market order on an exchange. You are querying for information, much like a seller is putting an intent to sell an asset. Google then looks through its database of information and surfaces cafes relevant to you. To rank the information it surfaces into a list, Google would consider your location, transaction history, reviews from locals and advertisement dollars from sponsors.
Like an exchange, the buy side of this order consists of firms that have paid to be on the list and have earned their spots there.
Given enough labour and computing power resources, large recommendation engines could be developed if the users have the intent. On social networks like X, formerly Twitter, matching engines (for advertisements) have never taken off at scale because the users’ intent is not to make purchases. It is to consume content. Erstwhile twitter optimised to distribute good content that could retain users for longer.
Platforms like TikTok became popular because of how sticky they could make a content feed with the very small amount of information a user passes on to the platform. Google has an incentive to balance how finely they match your query. Too many advertisements, and users would not trust them any more than they trust Craigslist today.
Contextualising content that is specific to you whilst balancing commercial interests is Google’s fine art. In some sense, they have become a matching engine where liquidity consists of human attention and indexed content from the internet.
Humour me for a bit longer. Much of the internet today functions like matching engines. Amazon matches consumers with product inventories. Instagram matches creators with an audience base. X matches users with regrets about going online. Tinder or Bumble matches users with potential partners, experiences or regrets. Surely, there are operational aspects to these businesses. But at their core, they match supply and demand, like a matching engine at an exchange.
Moats for these matching engines come from the proprietary data they own. For instance, Uber has the largest database of drivers in some cities. Airbnb has a closed database of properties: the reviews they receive and historical data of how many properties open up in each city at different seasons. The data is the secret sauce based on which businesses can monetise themselves in myriad ways. It is an asset developed through the scale these businesses have reached and the longevity with which they function.
I will resist the urge to go into large language models (LLMs) and AI for now, but my point is that the closed-off data sets these platforms have is their moat. Every once in a while, aggregators or platforms interact with one another. For instance, you can book an Uber from Google Maps in some regions.
So, interoperability of assets – be they drivers, products or inventories of houses – exists today. But they are owned by the businesses that maintain the inventory and can be shut off at will. The moats of these businesses exist in closed graphs that are privately owned. Quite recently, Reddit was mired in controversy for restricting API access to third-party applications that showed content from the platform.
Open Graphs
Smart contracts enable decentralised matching engines on the open graphs that blockchains are. Unlike data on centralised servers, blockchain data is accessible to anyone. Uniswap is a matching engine for assets to convert from one to another. Aave is a matching engine that holds an asset in exchange for giving another as a loan.
These matching engines work without human intervention or external data because their logic is pretty simple. Aave checks if a loan amount is collateralised for the right amount. Uniswap’s smart contract sends tokens to a pool and withdraws assets based on the AMM formula used.
These engines provide rich public data that providers like Nansen index. At scale, you can take data from matching engines like Aave and Uniswap to provide historical context. Labels on Nansen are assets developed using data from public matching engines. How do they work? You study a user’s behaviour over time to track their historical P/L. Wallets that were early to an NFT and held a token through a bear market or early to new smart contracts are considered ‘smart money’.
Historically, the demand side for such context came from traders who wanted to study other traders’ activities. Knowing a large fund is selling a token or using a smart contract provides a tactical advantage when managing millions of dollars online. So service providers have considered DeFi the core market for building context and data sets. I have written at length about this in The Data Wars.
The incentives to retain and continue using a wallet have been relatively low. For instance, you use the same Uber account for years because your reputation determines the quality and pace of service you receive. A low rating could mean worse drivers for you. On Airbnb, hosts check your past bookings to see if they’d want to open their house to you.
Even in games like GTA 5, being a bad player that goes around killing other players in a server (called griefing) gets you put in a server with other griefers. Your reputation holds value in the digital world, even when pseudonymous.
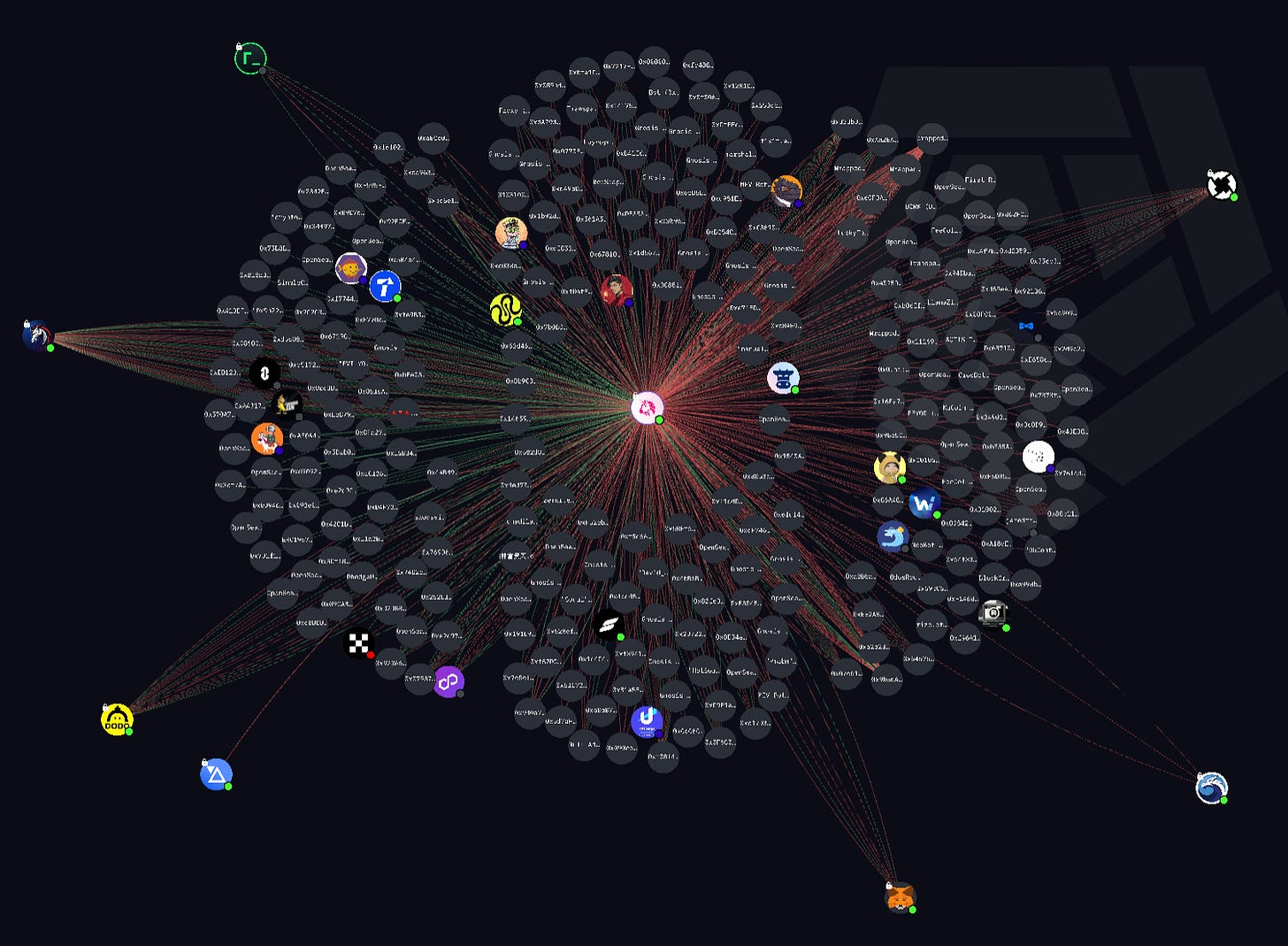
The incentive structures for blockchain applications have historically skewed towards anonymity for the average user. When spinning a new identity is as easy as clicking a button and there are no incentives to retain a wallet, we end up with many wallet addresses with scattered context. This is the crux of what limits Web3-native apps to grow beyond the crypto market. Applications in the industry face challenges in two unique ways.
Anybody can query a user’s historical behaviour with a product by querying blockchain data. No competitive advantage comes from privately storing information the way Meta or Alphabet does today.
Products generally lack context that goes beyond on-chain data about their users. Tools like ArcX are beginning to capture user information from browsers, but we are restricted in the nature of applications that can be built, as user information is often not captured.
Don’t get me wrong. There are some relatively novel solutions. For instance, Passport by Gitcoin allows a user to tie their real-life identity to a wallet. So, you could hypothetically have an AML-KYC’ed user on a perpetual exchange that uses a smart contract to match orders. Or you can use Worldcoin for a network of ~3 million users who have proved they are humans. In other words, the primitives we need to have a network of users with verified identities exist here and now today.
But you don't have much when you look at the supply side of applications that can cater to such users. Users have no incentive to verify their identity and be onboarding themselves.
To summarise :
Blockchain applications have historically been open graphs of economic interactions.
Smart contracts enable applications to become decentralised matching engines.
Users are not incentivised to add context to their economic activity on-chain.
Applications are restricted in what they can offer users due to the lack of context they have on users. This lack of context translates to an absence of moats among products built with open-source code especially if their communities have rallied around a token.
Due to this, the nature of the applications we build is optimised for low trust. Need a loan? Yep, you will need excess collateral for that. How about an asset swap? Sure, you need to be able to provide the exact amount for it. Wish to collect an item on a social network? Cool. Send in enough gas fees to mint the NFT and send it to your wallet.
The emergence of context at the periphery – through providers like Passport or Gitcoin – will soon enable a new generation of matching engines. And unlike DeFi or NFTs (which are huge on their own), this new generation of applications will potentially become what I consider ‘Internet-scale’. There will be a time when the use cases enabled by blockchain applications become relevant for the entirety of the web.
What would that look like, and how do we reach it?
Internet Scale
I was studying how transactional systems scale and saw a recurring pattern. Almost all of them initially focused on a dense network before they grew exponentially. Consider Visa, for instance. In the early years, they sent out some 60,000 unsolicited credit cards to a population of 250,000 in California.
By having ~25% of the population own credit cards in a region, they could convince some ~20,000 merchants to begin accepting Visa for payments. Keep in mind the geographical density of the consumers is what drove the merchants to adopt Visa. If the users were distributed worldwide, it would be like crypto today: too small a market for a merchant to care about.
When Stripe began making online payments easier, it started relying on Y Combinator’s network of startups to go from 0 to 1. This was despite being in touch with both Elon Musk and Peter Thiel as investors. Y Combinator helped the startup find its initial group of users.
You had a variation of this with Web2 native social networks, too. Facebook launched with a geographic focus on students from Harvard. Y Combinator was critical for Hacker News’ growth in the early days. Alexis Ohanian from Reddit admitted to creating fake profiles on Reddit during its early days to signal activity on the platform. Without a user base, focusing on specific niches and mimicking activity becomes crucial to attract and retain users.
Web3 user accounts are public by default, and payment information is easily available to everyone. Moats in the industry would come from social networks that can build additional layers of context on top of the transactional data. What would that look like? Much like how Visa and Stripe had to focus on geographical density, Web3 social networks would have to look at niche-based density to scale.
These use cases must appeal to a large user base without making an incumbent feel deeply entrenched. Think, for instance, of Google Maps—a large use case with no strong incumbents. (Yes, GPS existed, but mobile-based mapping was not free).
When you think of Web3 payment networks in comparison, most products struggle to differentiate themselves due to two factors:
The core payment experience on Ethereum or Solana is excellent on its own. Transacting through a centralised provider often feels worse off than just using a wallet for a stablecoin transfer.
Startups struggle to differentiate themselves if payments (or transactional products) are the only USP. This is partly why there is a sea of dead DAO tooling startups.
One instance of a business I noticed in the wild providing ‘value’ beyond payment settlements is Request Finance. It is a simple invoicing product that allows users to collect invoice payments in stablecoins. I find it interesting because the product also has a repository of vendors on top of publicly available payment data (from wallet addresses).
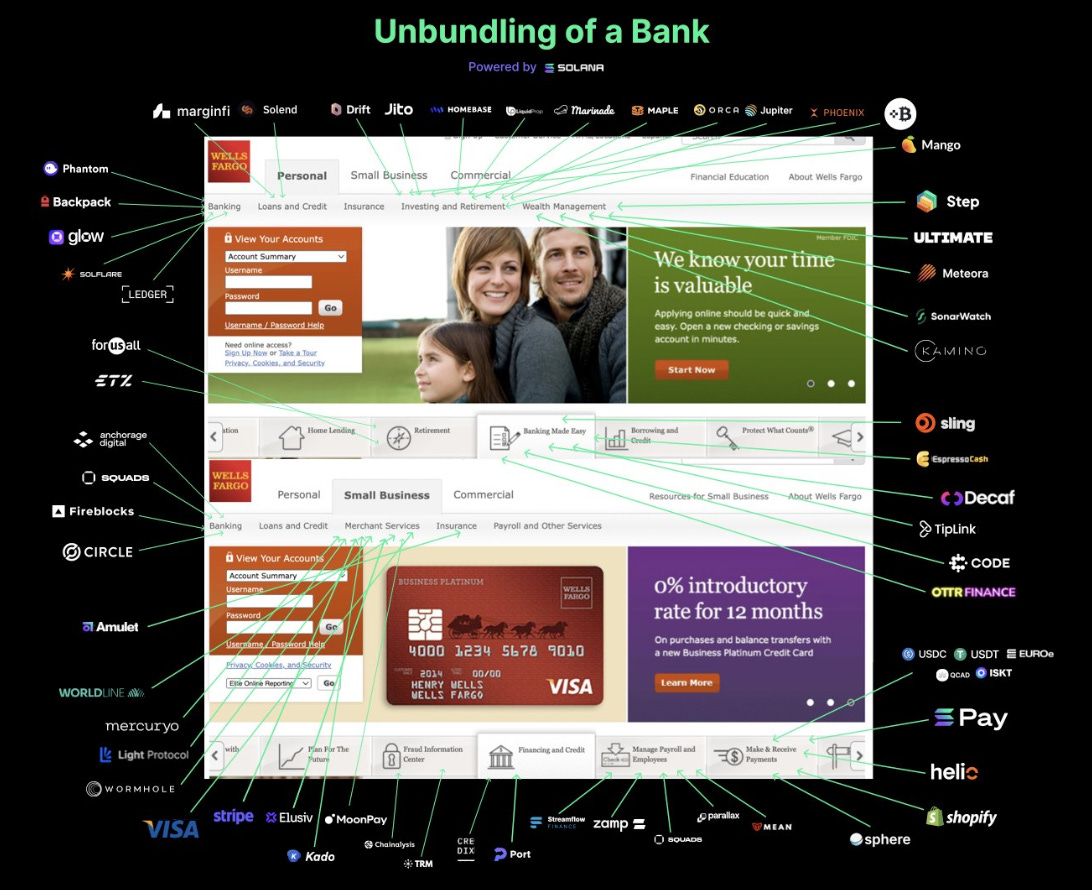
In such a case, blockchain data (of payments) with private context (on vendors and their relationships) built through providing a service (its invoicing product) helps the business develop a layer of context that is differentiated and unique to it. Whilst I’m not sure if the team plans on expanding to a marketplace, it is well within the possibility that they could build a repository of the best service providers, advertisers and DAOs using customer data they have access to.
In fact, they could even expand to offering lines of credit to platform users as they can see the frequency and amounts with which people are paid.
Combining user data from a blockchain and internal data sets from a service will likely enable the next generation of blockchain-native apps to scale. And much like we have with Web2, they will become matching engines at scale. In the example above, I presume that Request could expand into a marketplace model with a fintech component. However, most businesses are not there yet. The TAM of vendors and service providers in crypto is relatively miniscule.
Context Machines
Large businesses on the internet inevitably transit to enabling transactions on their products. Facebook, for instance, went from being a social network to having its own marketplace. In 2021, storefronts by the platform enabled 250 million users to transact with over a million shops. Apple went from being a hardware manufacturer to issuing its credit cards. This occurs because having context on user's financial behaviour makes it far easier to monetise the data you already hold on them.
As Saurabh wrote in our piece titled 'Zero to One', Web3 social networks might struggle to attract a critical mass of users because the incentives don’t exist for a normal user on the internet to port over. However, we have primitives that allow the identity of individuals to be verified far easily than on a Web2 platform.
For instance, a business that has context in the form of AML/KYC and financial history (from traditional sources like a bank) on a user, could offer undercollateralised loans to an individual. Today, when you use an app like Wally (a personal favorite), you give your spending habits to an app. What if it could be used to offer you credit from a protocol like Aave at better rates?
Collecting user data directly and mapping it to anonymous interactions on-chain can build better applications. I began working on this piece, wondering what it would take to make a better Web3 version of Tinder. I realised the use of open social graphs to enable dating goes back to 2013 when Hinge launched.
Dating apps are incentivised to retain users for the longest period because their revenue depends on it. Match.com would not be making $750 million every quarter if their userbase lived happily ever after with a few swipes.
But if we were to use Web3 primitives, one way to build a better dating app would be to
Capture user information in-app and through off-chain sources (such as Spotify, Instagram, Reddit and X)
Mapping social graphs to find friends of friends with shared interests and matching users with one another.
It sounds easy, but nobody is building it yet. The closest we have is a prediction market on Manifold. Speculating on whether a couple will stay together or not is, frankly, the most crypto-native outcome that could occur. Perhaps love - like many other human things, is not a problem for technology to fix. So, back to finance we go.
Combining on-chain transaction history and collecting off-chain data from users. If you use a Mercury bank account, you'll be offered invoice-based financing options to meet short-term credit requirements. The way the bank can offer you this is by keeping track of your revenue and matching you to external pools of capital. The bank itself has only one core asset: it's data on you. The service it provides is its ability to match you with a source of credit that can use that data.
As yields in DeFi dry up, platforms will emerge that tap into user data from off-chain sources and collaborate with on-chain liquidity pools to offer a higher yield. The difference between these tools and past versions of ‘undercollateralised’ lending would be the verification of user identity and the consequences of loan defaults.
This may seem far-fetched, but consider that both Maple Finance and Goldfinch service this function for SMEs today. They have privately held context through the data they collect from the users. They tap into a publicly available pool of money to underwrite and execute their loans.
A different place private context is built is in interfaces, such as websites or wallets. If you know a large number of users spend time on a certain kind of content or digital good, you can propagate it further to retain users. A new generation of content-related algorithm products, like MBD and Pond, are beginning to develop SDKs that make it easier to aggregate and create feeds of on-chain content.
But what if you could track user behaviour for how long they spend on certain content? Mirror’s team already curates stories on their landing page. This is one instance of a platform that can track how long users spend on each story to curate content.
In both instances, I presume that a public good – be it liquidity or content – can be better indexed and offered to niche users if you have private context as a product. But what incentives do businesses have to engage in such a model? It boils down to profit margins. Unlike Aave, a business lending to SMEs or individuals could demand a higher amount in yield.
A content aggregation platform that has scaled could (ironically) advertise products relevant for users. Unlike social networks of the past, a content aggregation platform relying on Web3 social graphs (such as the ones on Lens or Farcaster) would not have to maintain user databases or content. Their cost is in curating relevant content and capturing enough user data to be able to continue surfacing good content.
But how does such a system scale? App-chains offer clues. Last week, we wrote about dYdX. If you have an ecosystem of verified users (as on Worldcoin) or ones that bridged to your chain specifically to trade derivatives, you have drastically reduced CAC for newer DeFi projects looking to target users. Similarly, chains like Base, BNB and Kraken’s yet-to-be-launched L2 have a disproportionate amount of context on users as they already have data from their exchanges on each wallet.
It is kind of similar to the concept of agglomeration in economics. In bringing together large troves of users with similar interests, you unlock disproportionate amounts of economic activities compared to generic L2s, whose only edge is the speed at which they can enable a transaction.
This may seem far-fetched, but this version already exists within gaming today. Guilds like YGG are creating rich graphs consisting of users’ history on-chain. Game developers are incentivised to build for these users as they have rich, contextual data on their history. They could whitelist a handful of users who were early adopters of similar games and onboard them with incentives.
If you study the usage of Uniswap or Aave, it becomes pretty clear that Pareto laws apply to web3 native products, too. Building context on users is one more way businesses would accelerate the pace at which these laws emerge in our industry. So, are we repeating Web2 all over again? Not really. If settlements, social graphs and the content itself are on-chain, users cannot be deplatformed as quickly as they can be on Twitter or on a bank today.
In other words, the user can control their assets even when businesses build moats. The emergence of multiple clients - be it for seeking a loan or consuming content, would mean a user will have more alternatives than we do in a version of the internet where platform monopolies control our fates. For me, that is fundamentally exciting about the direction the internet is heading in.
Reading about Wikipedia,
Joel John
Thanks for the feature :)